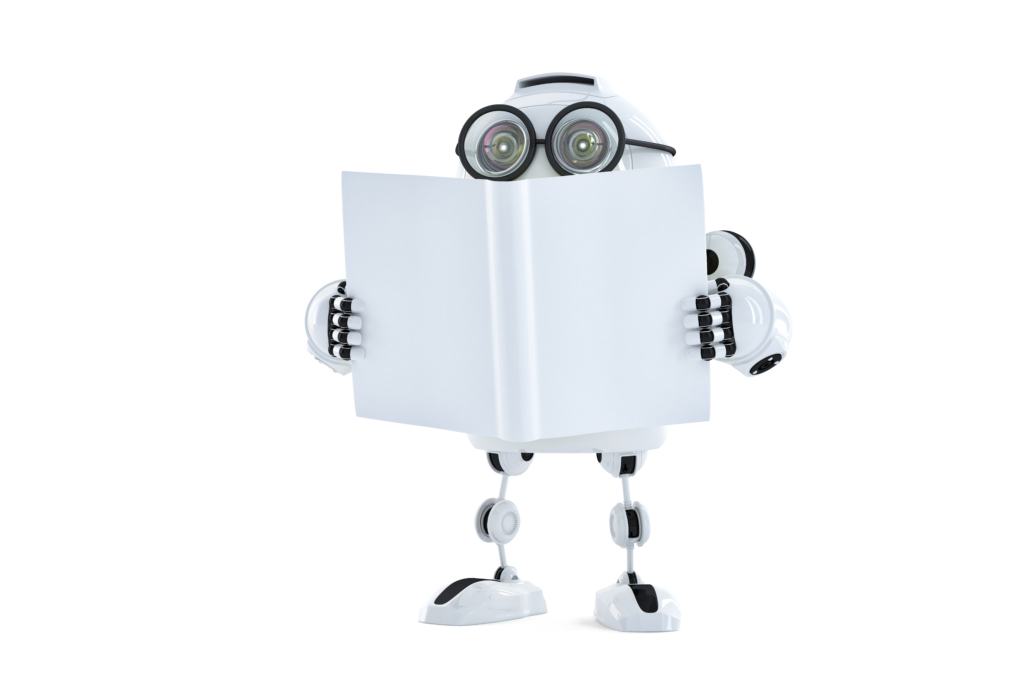
Data Literacy Is a Requirement for Generative AI Solutions
By Malcolm Chisholm
As Generative AI (GenAI) gets more and more popular, it is crucial for enterprises to begin to properly grasp true GenAI capabilities – especially as the hype and hopes around the technology grows. The LLM solutions are certainly breathtaking, but they have significant real-world limitations to be aware of. The biggest such limitation is data.
Never before have people been able to chat with data and get answers back the way they can now. It is certainly true that educating organizations about AI Literacy is essential as more and more GenAI projects get the green light. But the main driver of any Generative AI solution is data, as is clearly shown the technically complex data pipelines that get built in every GenAI project. Ultimately, an AI solution will only be as good as the data used to train it (we will use “train” here the broader sense, covering context windows, fine-tuning and RAG). Therefore, before we can even engage in AI literacy, we need to make sure there is an adequate foundation of Data Literacy.
Let’s go over one of the most basic scenarios – getting a complex data question answered. Without GenAI, this typically requires finding the right people, looking for the appropriate data assets, looking at BI reports, meetings, etc. This laborious process has historically been the only way for the business to get answers.
But if an enterprise has a high level of Data Literacy, they can speed things up substantially because they will have a greater understanding of the “who, what, where, when and how” of the people, processes and data assets involved. More advanced enterprises will have robust Data Catalogs in place, which act as a road map to the data, and will further optimize the discovery and understanding of data.
The goal of GenAI solutions is to get questions answered automatically, quickly and accurately. The above manual method has one major advantage over an automated search with an LLM chatbot. When people talk to other people, they can generally easily communicate or intuit context. In order for a LLM Q&A chatbot to properly function, context needs to be explicitly included in the training data. Properly accounting for nuance and context in data is crucial to any GenAI project, as the models should be trained with a specific purpose in mind. Without Data Literacy in place, everything gets very murky very quickly. There may be tempting sources of data in SharePoint Folders, Data Lakes, old Data Warehouses, and more, but how can we know if they are a match for the GenAI use case at hand? We need business professionals, who are very Data Literate, who can steer us to the right, accurate, timely, relevant data for a particular GenAI use case.
Unfortunately, if this is not done, then you can expect your GenAI solution to hallucinate, and give untrustworthy answers. It will not be the fault of the technology – it will be the fault of the data that was used. As stated before, context is king, and context will play a big role in the success of GenAI projects. The best way to make context explicit is for a GenAI project to work with Data Literate business professionals, who understand high quality, accurate, timely, and relevant data.
Once the foundation of Data Literacy has been laid down, and accompanied with a robust Data Catalog, then enterprises will have a much higher probability of successful GenAI projects.