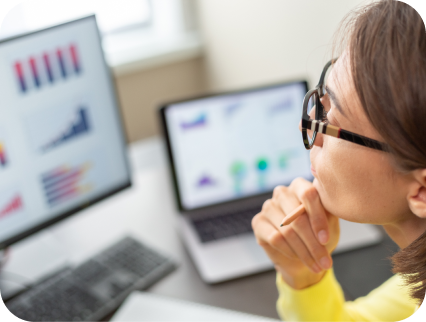
ANALYTIC MODEL GOVERNANCE
While Data Governance is focused on data as an enterprise asset, analytics is a very closely related area that also needs governance. We can think of analytics as consisting of data science, machine learning, with AI also being included, and all being grouped under “Model Governance”.
Model Governance differs from Data Governance in that it focuses much more on the model lifecycle, whereas Data Governance focuses on data management across the enterprise. That said, there are many capabilities of Data Governance that are needed for Model Governance, such as Data Quality and Information Knowledge Management. After all, models consume data and have to be documented. The methodology that Data Governance has to formulate and communicate policies and standards can also be leveraged by Model Governance for its own policies and standards.
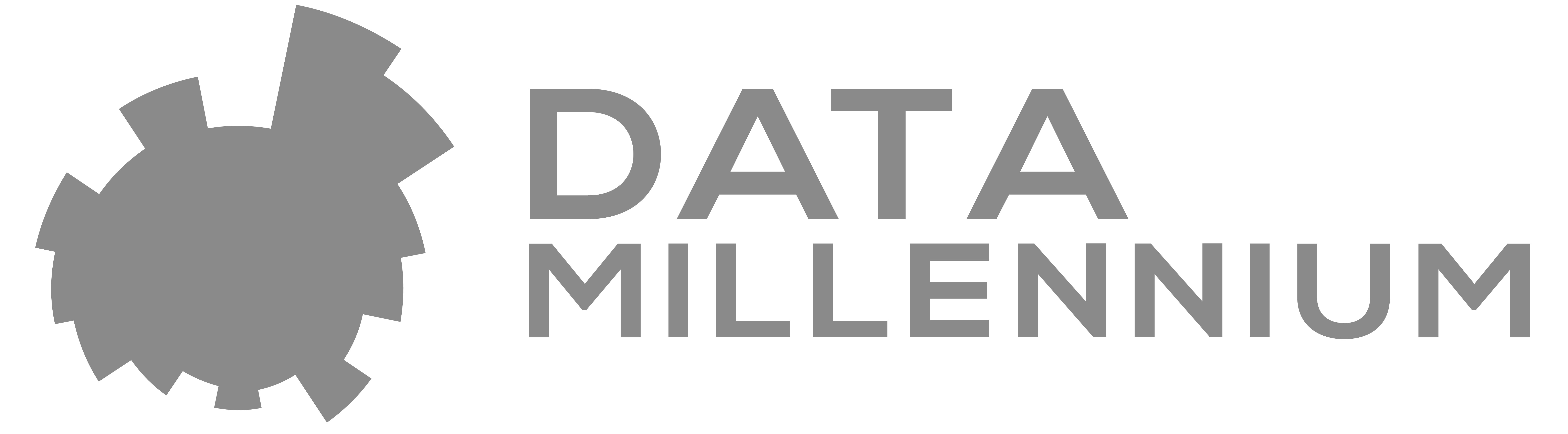
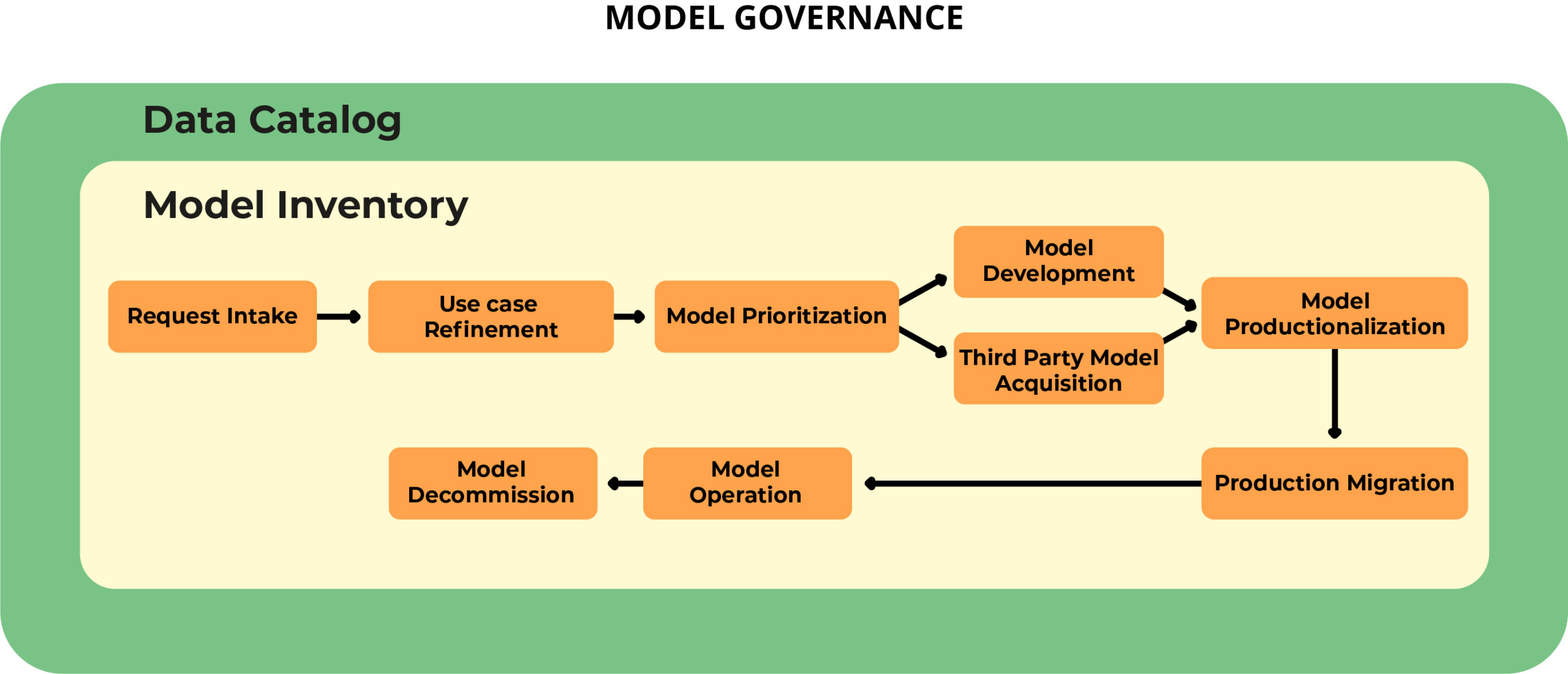
Fig 1. Model Governance is closely related to Data Governance. It differs in that it is built around the Model Life Cycle. A Data Catalog housing a Model Inventory can be very helpful in Model Governance.
That said, the model lifecycle has some unique requirements. Driving and managing demand is one. Models are developed in response to business requests, but the business has to be educated about what is possible and how to engage with the model developers. Regulatory and ethical concerns also need to be factored into a Model Governance operating model. Of particular concern is preparing a business unit for implementation of a model, which usually required change management and business process re-engineering.
Data Millennium has significant experience in building Model Governance operating models and programs.
CONTACT US
FOR MORE DETAILS ABOUT HOW WE CAN HELP YOUR ENTERPRISE BUILD THE CAPABILITIES NEEDED TO BE SUCCESSFUL.
Fig 2. Modern Data Catalogs have an ambitious scope. They automate the collection of often vast amounts of technical metadata. At the same time they support the needs of Data Governance to assign accountabilities and manage business metadata. They also increasingly play a role in active data management via workflows.
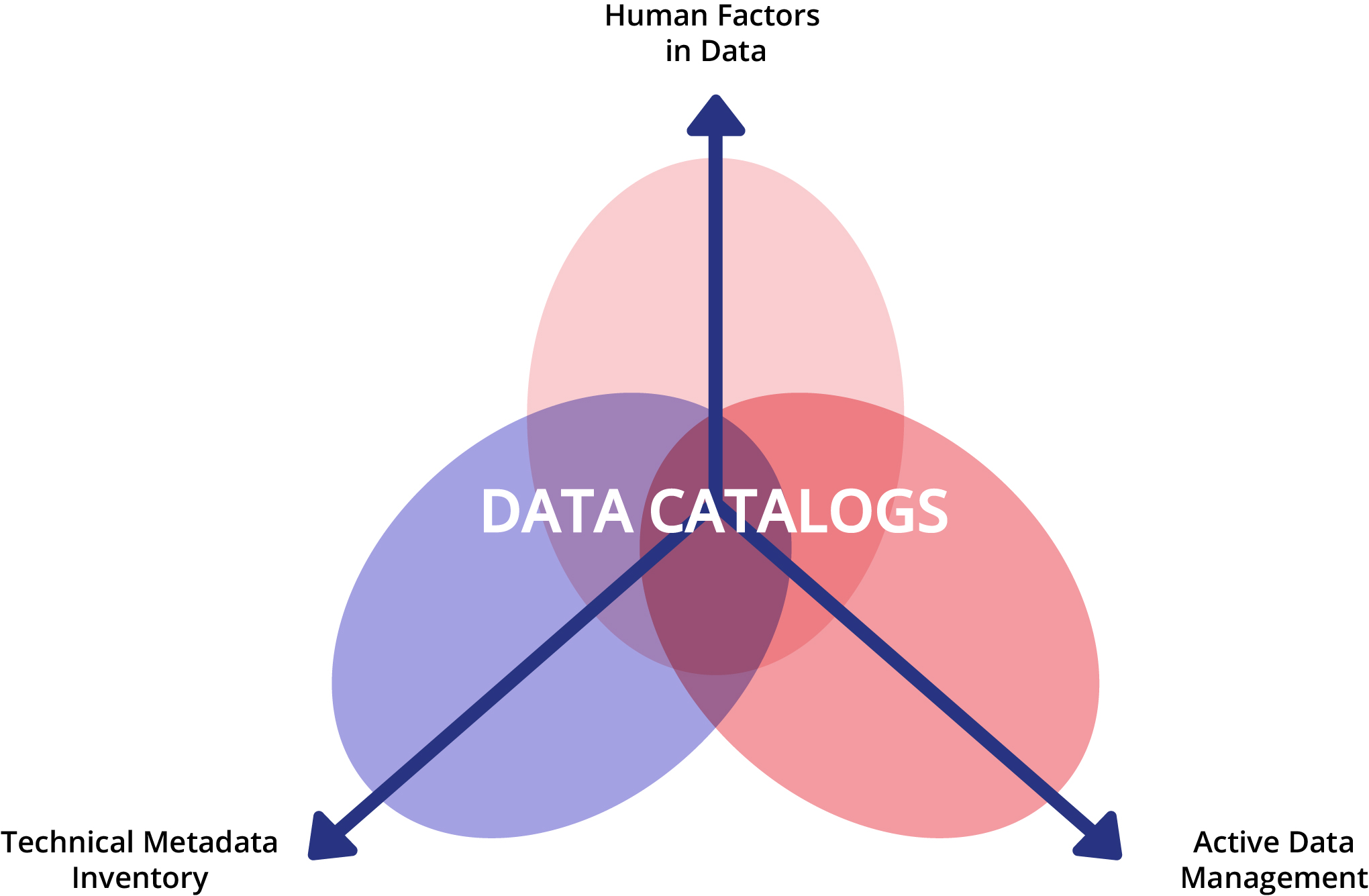
AI and Data Governance
With the advent of AI there is a growing realization that many new Data Governance tasks are required. There are, of course, many AI Governance needs, such as what LLM to select, and how to mitigate model risks. But even some of these overlap with Data Governance. For instance, AI models can be at risk if poor quality data is put into them.
In the context of AI, Data Governance tasks tend to follow the model life cycle. A lot of attention has to be paid to finding the right data to train a model, and ensuring this data can be used for AI needs. Data quality is of paramount importance. Sensitive data, like personal information, may have to be excluded from input to models, as will offensive language, and anything that might lead to bias. On the model usage side, prompt libraries, or meta-prompts may be required for the model to provide useful outputs.
To be successful with AI means engaging Data Governance to help with the data-related tasks for the models being built. AI is not simply technology into which any data in any format can be loaded. We can expect to see a much greater evolution of the symbiosis of AI and Data Governance over the next couple of years.
CONTACT OUR TEAM OF EXPERTS
Don't Hesitate To Connect With Us!
Please use this form and we will get back to you as soon as possible.