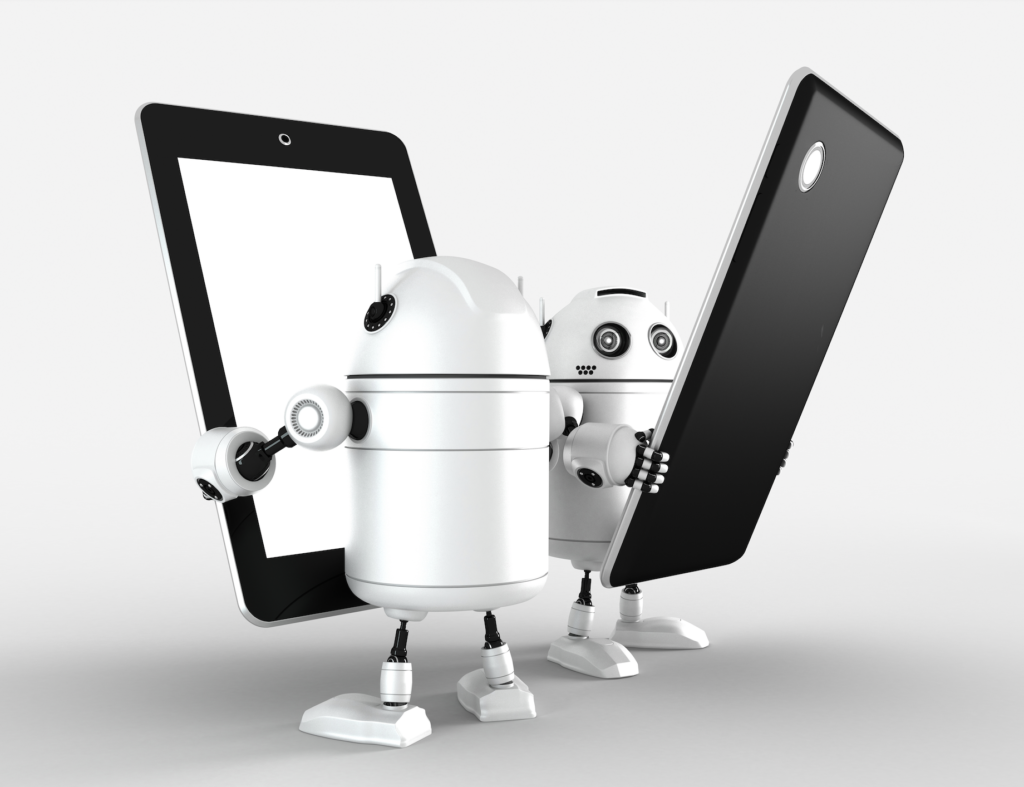
Is Everybody Speaking the Same Language – Even Your AI Agents?
By Malcolm Chisholm
Enterprises of all sizes face the issue of making sure the terminology across different groups or
departments is being used correctly and accurately. The classic example is the term
“customer”, which can mean very different things in different groups. Accounts receivable might
consider a “customer” as someone who made a purchase, and marketing might consider a
“customer” as the audience for a marketing campaign. The issues arise when folks from
different groups try to communicate to each other and don’t realize “customer”, while the same
word, has a different meaning. A Data Catalog is a great place to properly and thoroughly
define terms in a Business Glossary, so conceptual distinctions can be referenced and
understood.
Using GenAI tech, AI Agents can be trained from group/departmental data. An Agent can be
trained, prompted to answer questions with a certain style (aka: give it a personality) and then
become a 24/7 Q&A and solutioning resource. Unstructured documents are a great treasure
trove of learning potential for an AI Agent – however, terms and definitions will be implicitly
defined here. To make an Agent provide the best possible answers, a very well-structured
Business Glossary with explicit, thorough definitions is critical. With precious compute
resources being spent on an Agent, it is a bad practice to have an Agent infer definitions. By
the way, it is also suboptimal to have humans infer definitions too.
Let’s briefly review a few scenarios:
1) Human to AI Agent – A person from a different department asks an AI Agent what
formula is being used for a certain field in a report. From the report, the Agent should
know the context and be able to answer questions. But what happens if a question is
more abstract, such as why were more widgets sold versus gadgets? An Agent must
understand terms and concepts in the right context to provide an answer.
2) AI Agent to Human – As Agents eventually handle and even manage processes, an
Agent may reach out to a human for help answering a question. The Agent must
effectively and accurately use the right terms in context. For instance, an Agent may ask
a Data Steward about a Data Quality exception, and explain, in context, why certain
values are exceptions.
3) AI Agent to AI Agent – Agents are already working with each other to solve problems
on a small scale, and this will only increase. We can already see this with frameworks to
develop software. Like human-to-human communication, Agents must talk to each other
with a high degree of accuracy using correct terminology. This is necessary, otherwise
they will produce unworkable, broken, nonsensical solutions. To avoid communication
issues, concrete terms and definitions that each Agent has to work with are crucial.
In conclusion, Data Governance must help ensure AI Agents succeed with solid Business
Glossaries to stay relevant as AI evolves with these Agents. The Business Glossaries must of
course provide complete definitions including nuance, context and relations. Data Catalogs are
the best place to document, curate and maintain Business Glossaries, and Business Glossaries
in turn become an essential structured data source for Agents. There are right ways and wrong
ways to build and maintain a Business Glossary, and it is more nuanced with AI and knowledge
graphs.
If you want to have a more nuanced discussion, then let’s connect and see how you or your organization can build, maintain and curate Business Glossaries that are useful to emerging AI Agents.
contact@datamillennium.com